Capturing my evolving thoughts on GenAI & Datacenter capital expenditures
It is rapidly evolving and changing, always good to take stock of what is happening.
I aim to write more timeless than timely, but I thought this piece was needed to capture and clarify my thoughts and allow others to get a glance at how I am thinking about this evolving and rapidly changing technological developments of AI and GenAI.
From AI to GenAI. AI has been an ongoing development for the last few decades, whereas Gen AI is newer and is supported by Large Language Models (LLMs).
Right moment of hardware and software in 2022. The right moment for hardware and software really came together with OpenAI's ChatGPT launched in November 2022 supported by NVIDIA’s GPUs, and we saw one of the most rapid technological adoptions we have ever seen.
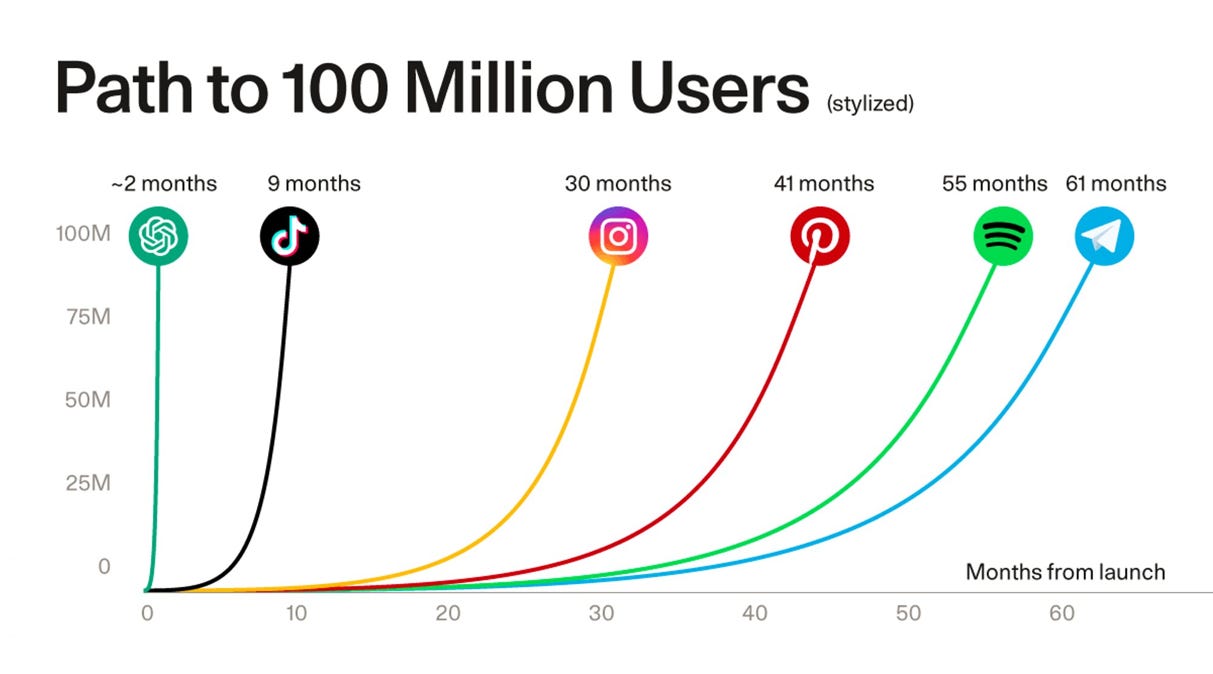
From general computing to parallel computing. We look to be in the initial buildout phases of transitioning from traditional CPU general computing to parallel GPU computing infrastructure to drive Artificial Intelligence (AI) and Generative artificial intelligence (GenAI) rollouts.
Still early in the S-curve ramp. We started with silicon first, then moved into infrastructure and platform. Next is moving into Models and Machine Learning (ML) Ops and into Software and Applications, then Services.
Hallucinations. Hallucination from LLMs and Gen AI is a feature, not a bug. It works incredibly well for creative work, like advertising and creative content creation. It does not work particularly well for highly repetitive tasks that require specific calculations and computations that require one to be correct. For example, Retrieval-Augemented Generation (RAG) techniques can enhance the accuracy and reliability of Gen AI models with facts fetched from external sources.
General purpose vs specific purpose chips. General-purpose chips like NVIDIA's GPUs for training and inference will work well for most customers. However, if a hyperscaler has sufficient scale and technological know-how, it can create specific chips for each type of workload and reduce its heavy reliance on NVIDIA. Amazon has Trainium for training and Inferential for inference. Google has its ASIC-based TPUs, Microsoft with the Microsoft Azure Maia AI Accelerator, optimised for AI and GenAI, and Microsoft Azure Arm-based Cobalt CPU. These chips are for running specific workloads, and specialisation can result in higher cost efficiencies and savings. Many others (e.g. Meta and Tesla) prefer to let NVIDIA do their thing best in designing chips, and they focus on using the chips and on their business.
Model improvements. When ChatGPT first came up, the innovation was brand new. It has since moved from text to pictures, videos, audio, songs, and more. The mediums will expand beyond our wildest dreams. The improvements will seem the largest initially, but becoming less and less incremental over time. LLM models could be commoditised over time, with increasing convergence driven by network effects, to an outcome where a few models will dominate. There will probably be a mix of closed-source (e.g. OpenAI's ChatGPT, Anthropic's Claude, Google's Gemini) vs open-sourced (e.g. Meta's Llama, Mistral).
Training vs Inference. I don't think it is an either-or. At the start, most of the workloads will be predominantly training (of the models), and the training workloads will shift more towards inference. There should always be training so the model can constantly take in new data to train and improve it further. At a steady state, workloads could well be 60-80%+ inference.
Use cases translating into ROI. Ultimately, the capital expenditure (capex) investments must translate into valuable use cases that create long-term sustainable economic value. The customer use cases are the most important. Would these lead to saving costs, increasing customer stickiness, or increasing revenues? These capex spending would ultimately translate into ROI; if not, the capex investments will stop. The returns might not come immediately now, but they should be clearer a few quarters or years later.
Use cases. Are the use cases from GenAI stronger than those from crypto, blockchain, web3, or De-Fi? I think there is significant potential. I think the most extensive use case is in advertising, which lies with content and data. Meta is one of the largest beneficiaries, as Mark Zuckerberg took a large calculated bet a few years back when Apple forced his hand with the iOS privacy changes.
Capex investments by the hyperscalers. Thus far, the hyperscalers are leading the multi-year capex investments. The hyperscalers are some of the biggest and most profitable companies, with the most robust balance sheets and the highest capability to invest these large amounts. They are in a catch-22. They need to invest. If they invest and it turns out well, they will do well. It will be horrid if they don't invest and lag behind their peers who do. Thus, it makes sense for them to invest as long as it does not destroy them if this does not pan out. They are thoughtful about the pace of investment and are seeing strong customer demand and using that as a leading indicator. They are also trying to be flexible and be able to redirect the GPUs to other parts of their business should customer demand eventually dissipate, which goes back to use cases and long-term value creation.
Human job displacement. White-collar jobs are most at risk, rather than blue-collar jobs, which require a lot of manual labour. Human productivity will increase and could displace repetitive high-value jobs (think software coders, lawyers and accountants), and the job landscape will vary.
Proliferation of content. As the internet brought down the marginal cost of software distribution to zero, similarly GenAI is bringing down the marginal cost of content creation to zero. There will be more content and noise than ever, and one must differentiate between the real thought leaders and the real McCoys.
Market structure and potential disruption. Currently, the GPUs are a near duopoly between NVIDIA and AMD, with NVIDIA having anywhere between an 80-90% market share and spending nearly ~US$10bn on R&D. That is a significant barrier to entry for new startups. The opportunity for supernormal profits will attract new competitors and wannabe disruptors, and this is a space worth watching. AMD, on the other hand, is playing catch-up and getting orders as a backup to NVIDIA. For now, the hyperscalers' capex is NVIDIA's opportunity, as it is shifting value from them to NVIDIA. That said, NVIDIA is also making them stronger in the long term.
Top dog. The thesis is that NVIDIA can keep being the top dog and could keep winning (for now), as its innovation cycle is significantly ahead of its competitors. While competitors are trying to play catch up to NVIDIA's latest product, NVIDIA is already hard at work on its next-generation faster and cheaper products. It is like someone running at the same pace trying to catch someone running quicker and are already ahead. Unless the leader NVIDIA stops innovating and slows down, that would be the most significant cause of concern.
Less concerned about the potential of a glut in the next few quarters. NVIDIA has historically had episodes of glut with its gaming GPUs and GPUs for crypto when the bubble burst, so it's imperative to watch this development closely. For now, High Bandwidth Memory (HBM) and Chip-on-wafer-on-substrate (CoWoS) packaging remain somewhat supply-constrained from now to 2025, and commentary from TSMC is that it is only likely from 2026 that it could reach a balance. Supply remains tight, so a glut seems unlikely for the next few quarters.
AI at the edge. GenAI can be slow and takes time, with the inference returning to the cloud and your device. Apple could be a big winner here because of its devices' moat and ability to monetise them across its devices and different apps. Similarly, Google has started to monetise Gemini across all its Google cloud products.
Gauging the potential winners. For now, Meta, Google, Amazon, Microsoft, and Apple, followed by ServiceNow and Palantir, are the companies I am most excited about. They are seeing large and growing end-customer demand and are delivering sustainable value with real-life use cases.
Have strong convictions, loosely held. This view remains highly fluid and is constantly evolving as new developments occur. That is what we have to do as investors: keep up with the times, understand it, have a view, and take a stance.
4 August 2024 | Eugene Ng | Vision Capital Fund | eugene.ng@visioncapitalfund.co
Find out more about Vision Capital Fund.
You can read my prior Annual Letters for Vision Capital here. If you like to learn more about my new journey with Vision Capital Fund, please email me.
Follow me on Twitter/X @EugeneNg_VCap
Check out our book on Investing, “Vision Investing: How We Beat Wall Street & You Can, Too”. We truly believe the individual investor can beat the market over the long run. The book chronicles our entire investment approach. It explains why we invest the way we do, how we invest, what we look out for in the companies, where we find them, and when we invest in them. It is available for purchase via Amazon, currently available in two formats: Paperback and eBook.
Join my email list for more investing insights. Note that it tends to be ad hoc and infrequent, as we aim to write timeless, not timely, content.